TAU Ellis Unit Scholar Jonathan Berant was invited to speak at the first ELLIS NLP Workshop
- TAUadmin
- Mar 15, 2021
- 2 min read
Jonathan's talk titled: "Improving Compositional Generalization with Latent Tree Structures" demonstrates how an inductive bias towards tree structures substantially improves compositional generalization in two question answering setups.
During the 24th-25th of February 2021 the first ELLIS NLP Workshop titled: "Open Challenges and Future Directions of NLP" was held via a virtual meeting platform. The new established ELLIS NLP program, which is led by Iryna Gurevych, André Martins, and Ivan Titov includes NLP fellows and scholars from 15 European institutions. One of the invited distinguished speakers is Tel Aviv University Ellis unit's scholar : Jonathan Berant, an associate professor at the School of Computer Science at Tel Aviv University and a research scientist at The Allen Institute for AI.
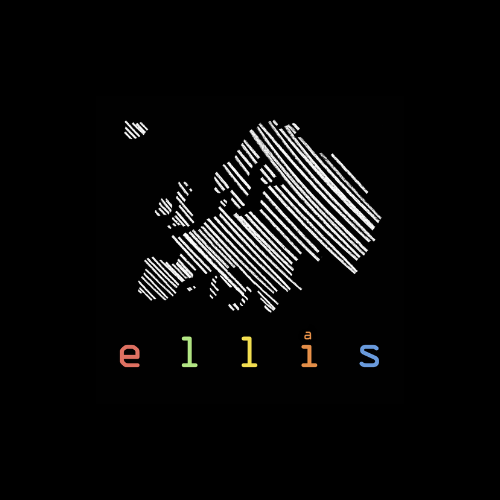
ELLIS (European Laboratory for Learning and Intelligent Systems )
A recent focus in machine learning and natural language processing is on models that generalize beyond their training distribution. One natural form of such generalization, which humans excel in, is compositional generalization: the ability to generalize at test time to new unobserved compositions of atomic components that were observed at training time. Recent work has shown that current models struggle to generalize in such scenarios. Jonathan presented recent work, which demonstrates how an inductive bias towards tree structures substantially improves compositional generalization in two question answering setups. First, by presenting a model that given a compositional question and an image, constructs a tree over the input question and answers the question from the root representation. Trees are not given at training time and are fully induced from the answer supervision only. The approach presented improves compositional generalization on the CLOSURE dataset from 72.2-->96.1 accuracy, while obtaining comparable performance to models such as FILM and MAC on human-authored questions. Second, a span-based semantic parser , which induces a tree over the input to compute an output logical form, handling a certain sub-class of non-projective trees. the presented work evaluates this on several compositional splits of existing datasets, improving performance, on Geo880 e.g., from 54.0-->82.2. Overall, these results are viewed as strong evidence that an inductive bias towards tree structures dramatically improves compositional generalization compared to existing approaches.
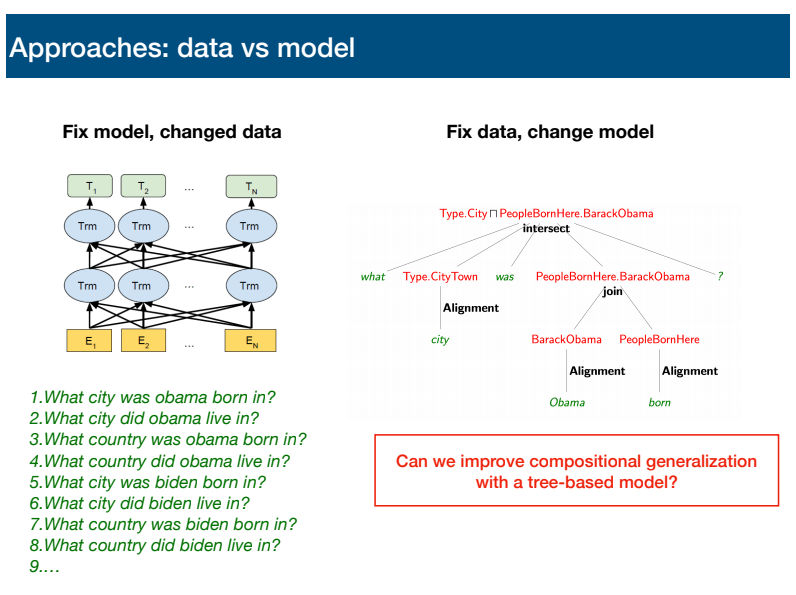
"Improving Compositional Generalization with Latent Tree Structures" ,Slide from Jonathan Berant talk at the ELLIS NLP Workshop, February 24 2021
Natural language processing (NLP) is transforming the way humans communicate with each other and with machines, with applications in multi-document summarization, machine translation, question answering, fact checking, decision support in health domains, or any applications which require making decisions that involve complex reasoning or entail combining different modalities (e.g., vision and text).
The goal of ELLIS NLP program is to facilitate collaboration across the leading NLP labs and to encourage closer interactions between the NLP and Machine Learning communities.
More information about ELLIS NLP Workshop (February 24-25, 2021) is available here .
More information about ELLIS is available here.
Comments